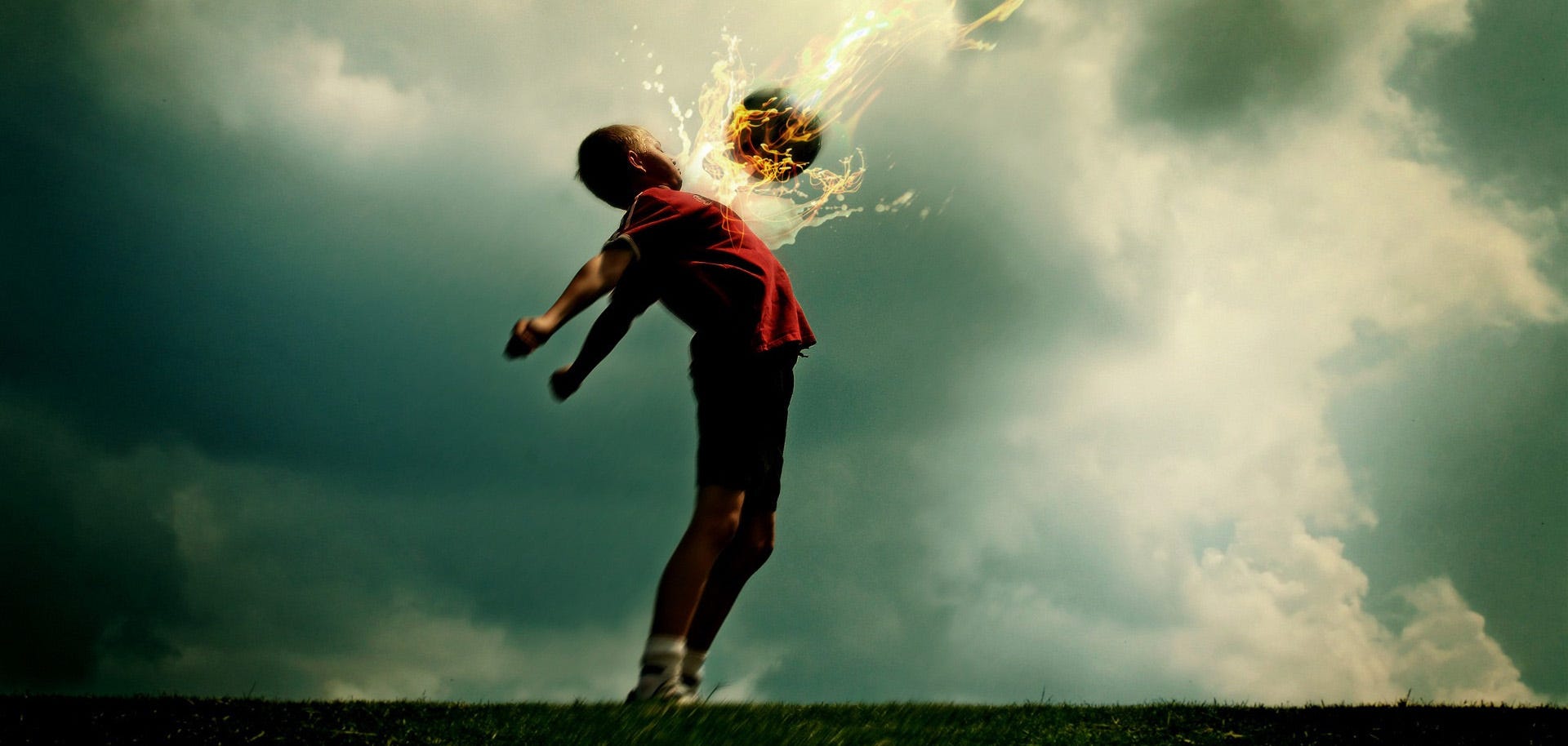
Your AI Superpower
8 reasons why epistemology, a largely ignored discipline within machine intelligence, is a must-have skill set for leading teams.
The goal of any artificial intelligence or machine learning system is to create good knowledge, whether in automating a process, predicting new observations, or illuminating entirely new domains. It follows that a fundamental consideration in machine intelligence is the underlying theory of knowledge creation. Just as flying machines are enabled through a theory of flight, knowledge creation machines are enabled through a theory of knowledge.
Just as flying machines are enabled through a theory of flight, knowledge creation machines are enabled through a theory of knowledge.
Obviously, a theory of knowledge isn’t an impediment to the pursuit of machine intelligence. People pursued flying machines long before a rigorous theory of flight was developed. Similarly, many are pursuing machine intelligence without a rigorous explanation of how good knowledge is created. Moreover, many successful practitioners would be hard-pressed to illuminate the theory of knowledge that underpins their solutions.
Epistemology is the branch of philosophy concerned with theories of knowledge. It’s knowledge about knowledge. As a stakeholder to a machine intelligence project, even an introductory understanding of epistemology is a superpower. But this proposition is a tough sell.
The skills deemed essential to machine intelligence are technical: computer science and mathematics, data analytics and statistics, software engineering and programming, as well as broad knowledge of the various technologies, tools and libraries that may be applied.
Which begs the question, why does your team need knowledge about knowledge? Here are 8 reasons:
- It’s unavoidable: knowledge creation machines need a theory of knowledge, just as flying machines need a theory of flight;
- Ignorance about the underlying epistemology enslaves people in methodologies;
- Epistemology levels the playing field for technical and non-technical team members;
- Epistemology provides an explanatory framework to more easily comprehend subject matter outside your expertise;
- Understanding epistemological controversies can combat indoctrination and stave off tribal infighting;
- Attending to an important and overlooked discipline can provide a competitive advantage;
- Epistemological theories can lead to new technology breakthroughs;
- Computational epistemology is a foundational consideration in the design of collaborative human-machine systems.
Let’s examine these benefits in more depth.
Epistemology is largely ignored in machine intelligence
While there is a general appeal in computing for softer skills from the humanities, philosophy is broadly ignored among machine intelligence practitioners. For many, “philosophical” means academic and impractical. As Freeman Dyson explained, “Philosophers became insignificant when philosophy became a separate academic discipline, distinct from science and history and literature and religion.”
Philosophers assume some of the responsibility for this state of affairs. Machine intelligence demands grounded theories that can be formalized and applied. There are philosophical schools of thought that are far removed from the pragmatic consideration of whether their theories can ever be instantiated in a machine.
But it’s folly to ignore the theory of knowledge underlying your project.
Are you empowered or enslaved?
It is impossible to avoid the epistemological concerns of your knowledge creation technology. As highlighted by Andrew Gelman and Cosma Rohilla Shalizi, “Philosophy matters to practitioners because they use philosophy to guide their practice; even those who believe themselves quite exempt from any philosophical influences are usually the slaves of some defunct methodologist.” If charged with the success of a project, the last thing you want to feel is enslaved.
“Those who believe themselves quite exempt from any philosophical influences are usually the slaves of some defunct methodologist.” — Andrew Gelman and Cosma Rohilla Shalizi
In the heat of a project, it’s entirely understandable that philosophy seems a distraction. But consider seriously the advice of Marcello Pelillo and Teresa Scantamburlo, which leads their excellent introduction to machine learning and epistemology:
As it often happens with scientific research, in the early days of machine learning there used to be a genuine interest around philosophical and conceptual issues, but over time the interest shifted almost entirely to technical and algorithmic aspects, and became driven mainly by practical applications. With this reality in mind, it is instructive to remark that although the dismissal of philosophical inquiry at times of intense incremental scientific progress is understandable to allow time for the immediate needs of problem-solving, it is also sometimes responsible for preventing or delaying the emergence of true scientific progress.
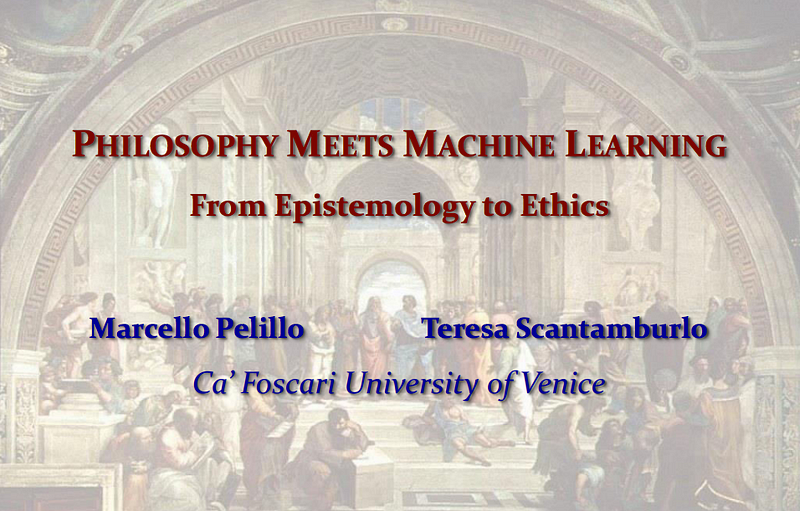
A level playing field for your team
Often times, the people that are ultimately responsible for the success of a project — executives, entrepreneurs, product leaders — become disenfranchised within their own projects. By making explicit the epistemological grounding for your project, you level the playing field and align expectations between the technical and non-technical team members.
Epistemology provides an explanatory frame for understanding difficult, highly technical subjects.
What I find most empowering about epistemology is that it provides an explanatory frame for understanding difficult, highly technical subjects in machine intelligence. In this frame, a theory of knowledge is analogous to a theory of biology. I have no expertise in biology, but I find biology comprehensible through the theory of biological evolution. Theory provides explanatory reach, and reach confers cognitive efficiencies.
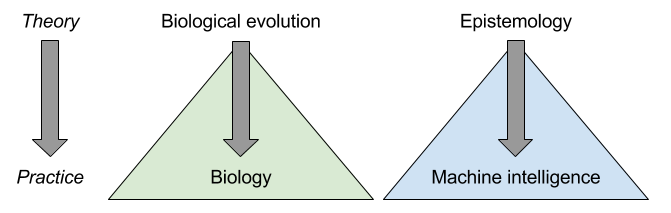
Similarly, a theory of knowledge empowers non-technical people with a frame for understanding and challenging technical machine intelligence practitioners. For example, epistemology explains why humans, for the time being, are essential to machine intelligence. It clarifies the limits, the breadth of application, for key technologies and mathematics. It illustrates how common sense and intuitive theories of knowledge creation can mislead.
This empowerment extends to technical team members as well. Given the explosion of interest and investment in machine intelligence, it has become impossible for any technical specialist to stay abreast of developments across the entire spectrum of concerns. By being attentive to the underlying epistemological assumptions and principles, you can quickly evaluate and assimilate disparate ideas within your area of expertise.
Epistemology combats indoctrination and tribal infighting.
Epistemological controversies are also creating fault lines and tectonic divisions between machine intelligence communities. If you are not explicit about these questions within your team, you’re undoubtedly carrying the risk of deep seated disagreements that can be counterproductive, even disastrous to your project.
In practice, you may not even recognize the root of these disagreements as questions of epistemology. Instead, they present as tribal affiliations and indoctrinations, like some perverse buzzword bingo, as opposed to questions for rational discourse. Believe me, you would much prefer rational discourse to tribal infighting.
Informing the future of machine intelligence
A discipline that’s revealed as both empowering and generally ignored can be a powerful competitive advantage.
We’re moving towards an inextricable merging between the technical manifestations of machine intelligence and epistemology, as computational epistemology.
In many ways we’re moving towards an inextricable merging between the technical manifestations of machine intelligence and epistemology, as computational epistemology. Machine learning and inductive reasoning comprise one example. In this respect, epistemology informs the very design of machine intelligence systems, particularly in the judicial use of both human and artificial intelligences.
There’s nothing soft about the intersection of epistemology and machine intelligence. It’s not only indispensable (and increasingly technical) knowledge, it’s a superpower for innovating around your competition, maintaining control over your project and empowering your team.
In this follow up post, we’ll explore a deep epistemological problem at the center of the AI landscape, and two opposing perspectives on how the problem is managed. This framework offers a simple yet far-reaching account of why AI works, the magnitude of the achievement, and where it might be headed.