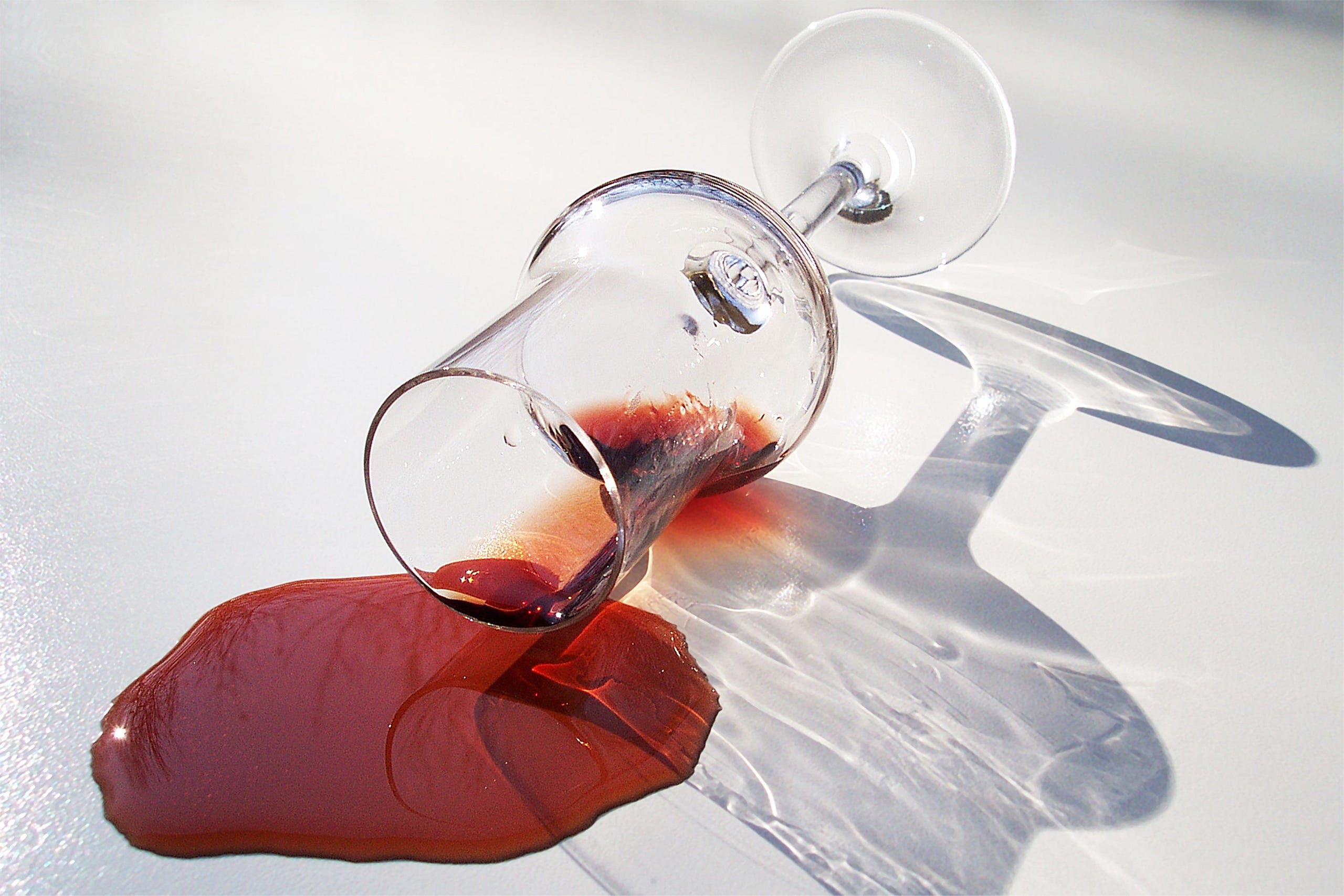
Medicine Under the Magnifying Glass
These detectives of research are shining a light on bad medicine. What are the implications for artificial intelligence in healthcare when many medical practices are wrong?
David Naylor, physician, researcher and former president of the University of Toronto, recently commented On the Prospects for a (Deep) Learning Health Care System. He highlighted a broad range of success stories: gene sequencing, computerized enhancement of medical images, clinical records and knowledge management systems, implantable cardiac defibrillators, sophisticated ICU monitoring systems, and “the endless variety” of automated processes for procurement, scheduling, and drug ordering. Deep learning promises to expand the range of applications considerably, from a beachhead in image analysis (including radiology, radiotherapy, pathology, ophthalmology, dermatology, and image-guided surgery), to new applications in risk assessments, logistics, quality management, and financial oversight.
Phew. It’s hard to behold such a breadth of “proven useful” applications and imagine that some significant problem is being neglected. I want to persuade you that this is indeed the case. Moreover, this problem has such reach that it imposes risks across the entire spectrum of use cases.
The problem is that a considerable portion of knowledge and practice in mainstream medicine is wrong.
Typically, when I introduce this problem to the uninitiated, I’m met with a sort of incredulity reserved for quacks. To deflect your concerns, let me introduce a few of the highly respected people that are tackling this problem from within medicine.
John Ioannidis is the main protagonist in our story. He’s a Professor of Medicine, Health Research, and Statistics at Stanford University, co-director of the Meta-Research Innovation Center at Stanford (METRICS), one of the most-cited scientists in clinical medicine, and was recently elected to the National Academy of Medicine for his seminal work in meta research. Vinay Prasad is a hematologist-oncologist and Associate Professor of Medicine at Oregon Health and Science University. And Adam Cifu is a general internist and Professor of Medicine at the University of Chicago.
In 2012, they offered this succinct summary of the problem:
How many established standards of medical care are wrong? It is not known. Medical practice has evolved out of centuries of theorizing, personal experiences, bits of evidence, expert consensus, and diverse conflicts and biases. Rigorous questioning of long-established practices is difficult. There are thousands of clinical trials, but most deal with trivialities or efforts to buttress the sales of specific products. Given this conundrum, it is possible that some entire medical subspecialties are based on little evidence. Their disappearance probably would not harm patients and might help salvage derailed health budgets.
In Part 1, I’ll introduce the problem of bad medicine. As you review the evidence for it, you’ll also get a good sense of why it’s so entrenched. In Part 2, I’ll examine the implications of the problem on artificial intelligence.
Part 1: How much of medicine is wrong?
“Half of what you’ll learn in medical school will be shown to be either dead wrong or out of date within five years of your graduation” David Sackett
In his history of Bad Medicine, David Wootton argued, “We can only think about medical progress if we start with the long tradition of medical failure.” We’re on a 2300 year journey from barbaric practices to modern science, from nonsense such as the ancient theory of the four humors and bloodletting, to medicine’s incomplete claim to empirical science today. As we’ll see, a good deal of medical practices have yet to be corroborated through rigorous experimentation and physicians can be remarkably slow to jettison old ideas.
So if medicine used to be all wrong, how much of it is presently right?
David Sackett, a founder of evidence-based medicine, famously said, “Half of what you’ll learn in medical school will be shown to be either dead wrong or out of date within five years of your graduation; the trouble is that nobody can tell you which half–so the most important thing to learn is how to learn on your own.”
His words seem intentionally hyperbolic, a wild estimate to reinforce the ethic of constant learning. But some take the estimate of half wrong very seriously.
In the prestigious medical journal The Lancet, its editor-in-chief Richard Horton made the case against science. He argued that “much of the scientific literature, perhaps half, may simply be untrue.” He offered an explanation that we’ll unpack below. “Afflicted by studies with small sample sizes, tiny effects, invalid exploratory analyses, and flagrant conflicts of interest, together with an obsession for pursuing fashionable trends of dubious importance, science has taken a turn towards darkness.”
Horton was expressing concerns reflected in many years of meta research (research on research). Most concerningly, he spoke of a culture of paranoia and secrecy. “The good news is that science is beginning to take some of its worst failings very seriously. The bad news is that nobody is ready to take the first step to clean up the system.”
I’m an optimist, so let’s focus on the good news, the serious efforts to understand the problem from biomedical research through to clinical practice.
A survey of meta research
Would you accept the validity of a criminal investigation if the investigators tampered with evidence? What if the investigation was politically motivated or the accusers had an axe to grind? What if there weren’t any independent corroborating witnesses or hard evidence? Similarly, meta research scrutinizes the environments and processes under which scientific research is generated.
John Ioannidis is perhaps its most celebrated and prodigious investigator. In a highly influential paper from 2005, he explained Why Most Published Research Findings Are False. “It can be proven that most claimed research findings are false.” His analysis was based on characterizations of research environments, using factors such as the prior probability of the study being true, its statistical power, and the level of statistical significance. Ioannidis concluded, “The majority of modern biomedical research is operating in areas with very low pre- and post-study probability for true findings.”
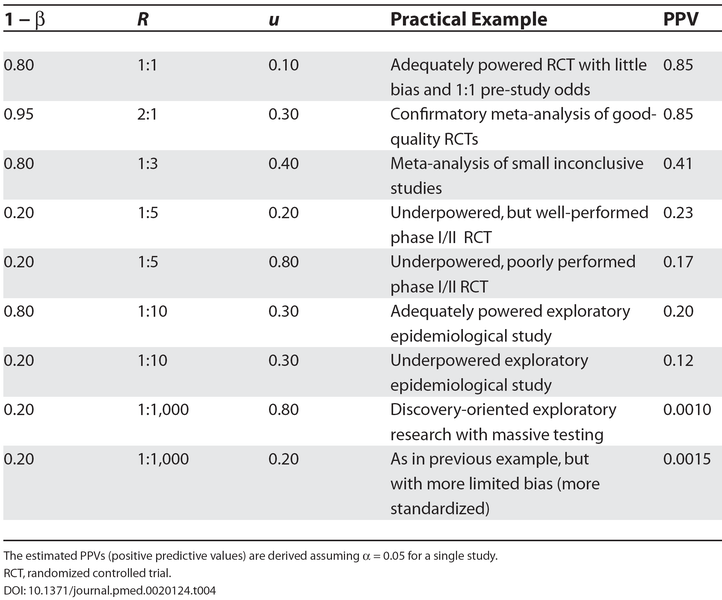
Reproducibility is another important measure of evidence. In 2015, Leonard Freeman and colleagues from Boston University investigated The Economics of Reproducibility in Preclinical Research. Their calculus incorporated a shocking rate of irreproducible studies. “An analysis of past studies indicates that the cumulative (total) prevalence of irreproducible preclinical research exceeds 50%, resulting in approximately US$28,000,000,000 (US$28B)/year spent on preclinical research that is not reproducible — in the United States alone.”
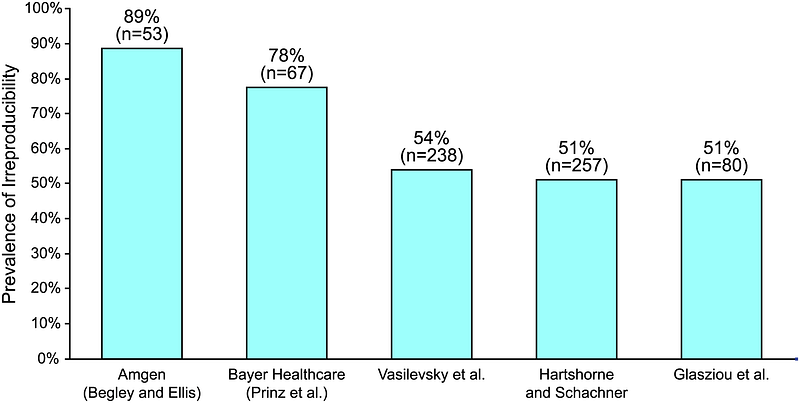
Criticisms of study design and reproducibility are like smoke. They don’t necessarily mean the purported research is wrong. For evidence of fire, we need to look at specific drugs, devices and procedures.
When researchers from the Ottawa Health Research Institute led by Kaveh Shojania investigated how quickly systematic reviews go out of date, they found that “new evidence that substantively changed conclusions about the effectiveness or harms of therapies arose frequently and within relatively short time periods. The median survival time without substantive new evidence for the meta-analyses was 5.5 years.” Surprisingly, research starts to depreciate as soon as you drive it off the lot. “Significant new evidence was already available for 7% of the reviews at the time of publication and became available for 23% within 2 years.”
Perhaps you feel this breakneck rate of change in medicine is a virtue, or at least a natural consequence of how “messy science” works. Unfortunately, Vinay Prasad and Adam Cifu contend that much of the change in medical practice isn’t a deepening of knowledge but the reverse.
In their 2015 book, Ending Medical Reversal, they explained, “Instead of the ideal, which is replacement of good medical practices by better ones, medical reversal occurs when a currently accepted therapy is overturned — found to be no better than the therapy it replaced.” They chronicle a disturbingly high number of examples. “We have seen that reversal occurs in all aspects of medicine. Whether the medical intervention is a pill, a procedure, a surgery, a diagnostic test, a screening campaign, or even a checklist that doctors or nurses follow, all sorts of medical practices have been found not to work.”
Based on their analysis of contradicted medical practices published over a decade (2001–2010) in the New England Journal of Medicine, Prasad and his colleagues found that 40% of the articles that tested an established practice reversed that practice, about equal the rate at which practices were reaffirmed as effective. (The remaining 20% are inconclusive.) They referenced a project of the British Journal Clinical Evidence, where a review of 3000 medical practices concluded that 35% are effective, 15% are harmful or neutral, and 50% have unknown consequences. “Our investigation complements these data and suggests that a high percentage of all practices may ultimately be found to have no net benefits.”
Practices that lack evidence survive in clinical guidelines, the recommendations clinicians consult in the care of patients. For example, a 2009 analysis of cardiovascular practice guidelines of the American College of Cardiology (ACC) and the American Heart Association (AHA) found that only 11% of the recommended practices conformed to the highest standards of evidence and 48% the lowest. Pierluigi Tricociand his colleagues concluded, “Recommendations issued in current ACC/AHA clinical practice guidelines are largely developed from lower levels of evidence or expert opinion. The proportion of recommendations for which there is no conclusive evidence is also growing.”
The net impact of medicine
Even in light of these studies, the problem of bad medicine seems fantastically implausible. If many interventions have no net benefit, you would expect the aggregate impact of medicine to be near zero. The bad would almost exactly offset the good. Are we really at a point where the net benefit of medicine hovers around zero?
“In the aggregate, variations in medical spending usually show no statistically significant medical effect on health” Robin Hanson
Robin Hanson, the economist and “father of prediction markets,” argues this case. Unlike factors such as exercise, diet, sleep, smoking, pollution, climate, and social status, Hanson maintains there is no correlation between medical treatment and health. In 2007, Hanson proposed a fairly blunt remedy: cut medicine in half. He acknowledged this isn’t “politically realistic” but he dares you to disagree with his diagnosis. “In the aggregate, variations in medical spending usually show no statistically significant medical effect on health. (At least they do not in studies with enough good controls.) It has long been nearly a consensus among those who have reviewed the relevant studies that differences in aggregate medical spending show little relation to differences in health, compared to other factors like exercise or diet.”
John Bunker, another pioneer in evidence-based medicine and the founder of the department of anesthesia at the Stanford University School of Medicine, arrived at a more charitable conclusion. His 1995 study found that medicine matters after all. “The extraordinary increase in life-expectancy that occurred early in this century has been attributed largely to non-medical factors. Life-expectancy has continued to rise, and medical care can now be shown to make substantial contributions. Three of the seven years’ increase in life expectancy since 1950 can be attributed to medical care. Medical care is also estimated to provide, on average, five years of partial or complete relief from the poor quality of life associated with chronic disease.”
A study of infectious disease mortality in the US during the 20th centuryled by Gregory Armstrong makes the observations of Hanson and Bunker more concrete. Most of the decrease in mortality over the 20th century is attributable to the sharp decline in infectious disease (with notable exceptions, such the pronounced spike of the 1918 influenza pandemic). From about 1950, the mortality rate continued to drop, but at a much more gradual rate. We’ve transitioned from high rates of infectious disease to high rates of chronic disease.
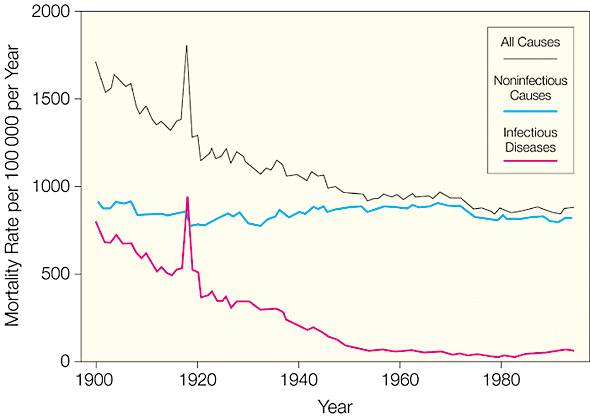
Medicine is certainly improving our lot in life, but the recent impact is less dramatic than people might imagine. Wootton concluded, “it is easy to exaggerate the extent to which medicine matters, but it would be strange to claim that it achieves nothing of any significance.”
We’ve moved from a beloved old adage to formal characterizations of systemic problems; from models that describe the unreliability of research environments and the impermanence of facts, to direct measures of medical reversals and under-evidenced interventions. We’ve reviewed the aggregate impact of medicine to consider the implication that, if a significant portion of treatments are ineffective, then we would see only a modest net benefit from medicine. And this is indeed what researchers find.
To restate, “How many established standards of medical care are wrong? It is not known.” But disturbingly, the estimate of half wrong cannot be dismissed as mere hyperbole.
If science has taken a turn towards darkness, efforts in meta research should be celebrated as beacons of light.
But it’s not enough. Hanson calls for action. “We already knew some medicine is more helpful and some more harmful than average. It doesn’t really help to know which part is which unless we are willing to somehow act on that information, and treat better parts differently from worse parts.” Ioannidis is similarly dissatisfied with problems. “My wish is not to expose problems. Obviously, there are lots of problems, so it is not a big deal to point out another one, but there is no end to them. My wish is to try to fix problems.”
In this spirit we turn to artificial intelligence. Is AI submerged in the problem, amplifying both good and bad medicine? Or might the AI community join the contrarians, disrupting medicine from the outside?
Part 2: Meta research and artificial intelligence
“It’s hard to be a disruptor in this area” David Moher
As Ioannidis highlighted in a recent interview with Eric Topol, the potential for meta research is astounding. “One-percent improvement because of adopting a better scientific process across science is tremendous progress. It could translate to tens of millions of lives saved.” Given the trillion dollar scale of healthcare and the proportion of questionable medical practices, it could also save billions.
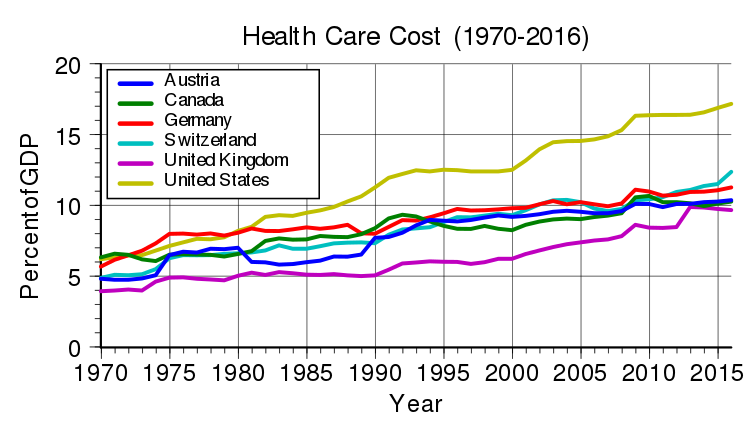
Do these lives and riches translate to substantial investment in meta research? Unfortunately, not yet.
Meta research as a field
As reported by Jennifer Couzin-Frankel in Science, funding for people working within meta research is scarce. “If you approach government funders, foundations, often they’re asking what diseases you’re curing,” says An-Wen Chan, a skin cancer surgeon and scientist at Women’s College Research Institute in Toronto. Funding is typically organized along topical lines, not cross-disciplinary methods. “Research in this area is not fast-moving,” says Sara Schroter, a senior researcher at The BMJ. “It’s hard to be a disruptor in this area.” according to David Moher, a clinical epidemiologist at The Ottawa Hospital Research Institute.
In one sense, efforts in meta research are ubiquitous. Scientists from all fields are continually honing their methods and processes. But these efforts are also highly fragmented. Coordination and knowledge-sharing is limited.
In their review of the meta-research field, Ioannidis and his colleagues called for a more unified effort. “A research effort is needed that cuts across all disciplines, drawing from a wide range of methodologies and theoretical frameworks, and yet shares a common objective; that of helping science progress faster by conducting scientific research on research itself.”
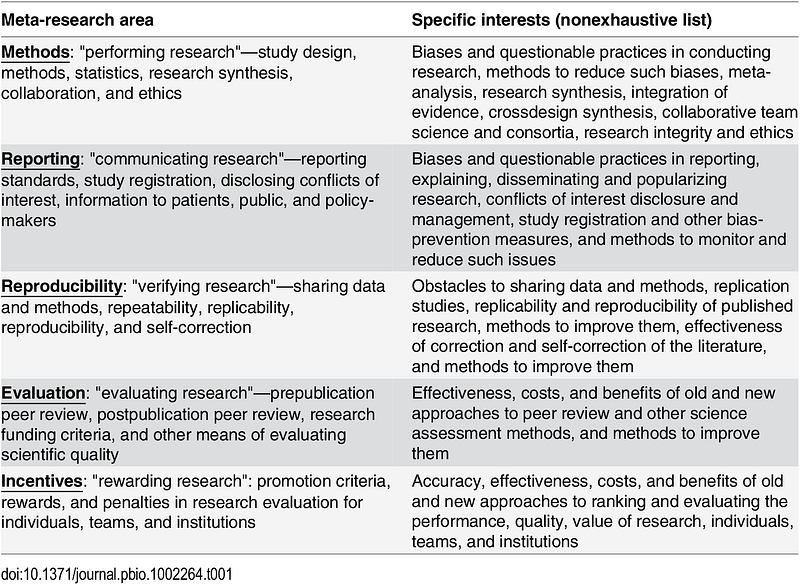
Dominant categories of AI investment
Meta researchers are increasingly using AI and machine learning techniques to increase their productivity. A notable example is the recent work of Thomas Stoeger and his colleagues at Northwestern University. They investigated why biomedical research focuses on a minority of all known genes. “Our work demonstrates that even highly promising genes that could already be studied by current technologies remain ignored.”
Their research drew international headlines, highlighting a problem that would otherwise fester in darkness. Such is the promise of AI as a searchlight for meta research.
But meta research has not yet been widely embraced by the AI community at large. The dominant categories are focused on applications within the existing medical and healthcare complex.
According to principal analyst Keith Kirkpatrick of the market intelligence firm Tractica, “Cost reduction is a major driver of many healthcare initiatives and incorporating AI technology is no exception. AI applications are designed to address specific, real-world use cases that make the diagnosis, monitoring, and treatment of patients more efficient, accurate, and available to populations around the world.”
While ROI and cost reduction drive investment, it’s hard to connect the dots in meta research, from abstract processes and methodologies to the massive costs of over-diagnosis and over-treatment.
Ironically, most of the AI community is focusing its productivity punch in areas that are already being criticized for moving too fast. While medicine is accelerating, so is the prevalence of under-evidenced treatments. This is hardly surprising, given that only a small fraction of funding is directed to treatment evaluation (less than 10%, based on a 2006 review of UK health research funding).
AI is mirroring existing investment patterns, incentivized to produce more medicine at lower costs. If data is the new oil, too much medicine is runaway climate change.
And the window of opportunity may be closing. The applications for AI in healthcare have been “proven useful” and are thus crystallizing. Startups and investors are defining the product-market boundaries for AI and healthcare, as a necessary precursor to commercialization. While their optimism and commitment are cause for celebration, it’s also important to note that these categories establish not only what will be pursued, but also the areas at risk of neglect.
Is AI is necessarily good for medicine?
Some might argue that all efforts in AI tackle the problem of bad medicine. There’s a widespread belief that knowledge can be derived from data, and that machines can overcome the inherent biases and failings of human decision-makers. If true, bad medicine isn’t an impediment to AI, it’s what makes AI necessary.
It’s a big question, so I’ll return to it in a future post. For now, suffice it to say skepticism is warranted.
Garbage in, garbage out is a principle of computing as old as Charles Babbage. As discussed in a previous post, the main challenge with today’s AI concerns this very problem of how to manage the problem of induction to create good knowledge. Inductive methods require sound explanations and background knowledge to work effectively. There is no universal learner that works well in all environments, so existing domain knowledge is an essential factor.
And there’s no shortage of contemporary examples of algorithms amplifying bad choices, from racist chatbots, to discriminating recruiting systems, to unethical assessments in legal proceedings.
AI offers no all-purpose cure. A fundamental problem like bad medicine needs targeted solutions.
A new calling for AI
In his 2016 confession to David Sackett, Ioannidis offered a hand-wringing testimony of his experiences in clinical research. “David, I am a failure.” He described a deteriorating problem. “The GDP devoted to health care is increasing, spurious trials and even more spurious meta-analyses are published at a geometrically increasing pace, conflicted guidelines are more influential than ever, spurious risk factors are alive and well, quacks have become even more obnoxious, and approximately 85% of biomedical research is wasted.”
“David, I am a failure” John Ioannidis
It’s painful to hear champions like Ioannidis describing their efforts as failures. It takes courage to tackle this problem from within. But the existing incentive structures in healthcare make meta research an uphill battle for incumbents.
This is why meta research needs more attention from startups, technologists, and investors.
Healthcare is an inhospitable environment for innovation under the best of circumstances. Those that choose to innovate in this area are trying to make a difference, to save lives and improve human well-being. The data scientist, Benjamin Rogojan, captures the spirit of this higher purpose well. “As healthcare professionals and data specialists, we have an obligation not just to help our company, but to consider the patient. We need to not just be data driven but be human-driven.”
Meta research offers the AI community a powerful opportunity to be human-driven. It embraces perhaps the biggest problem in healthcare. “Move fast and break things” doesn’t play well in medicine, but this is the meta realm. Meta research needs your speed. It needs your urgency. It not only welcomes disruption, it can’t succeed without it.
Armstrong, G., Conn, L., Pinner, R. (1999). Trends in Infectious Disease Mortality in the United States During the 20th Century. JAMA. 1999;281(1):61–66. http://doi.org/10.1001/jama.281.1.61
Bunker, JP. (1995). Medicine matters after all. Journal of the Royal College of Physicians of London. https://www.ncbi.nlm.nih.gov/pubmed/7595883
Couzin-Frankel, J. (2018). Journals under the microscope. Science. http://doi.org/10.1126/science.361.6408.1180
Freedman LP, Cockburn IM, Simcoe TS (2015) The Economics of Reproducibility in Preclinical Research. PLoS Biol. https://doi.org/10.1371/journal.pbio.1002165
Hanson, R. (2007). Cut medicine in half. Cato Unbound. https://www.cato-unbound.org/2007/09/10/robin-hanson/cut-medicine-half
Horton, R. (2015). Offline: What is medicine’s 5 sigma? The Lancet. https://doi.org/10.1016/S0140-6736(15)60696-1
Ioannidis, JPA. (2005). Why Most Published Research Findings Are False. PLoS. https://doi.org/10.1371/journal.pmed.0020124
Ioannidis, JPA. (2013). How Many Contemporary Medical Practices Are Worse Than Doing Nothing or Doing Less? Mayo Clinic Proceedings. https://doi.org/10.1016/j.mayocp.2013.05.010
Ioannidis JPA. (2016). Evidence-based medicine has been hijacked: a report to David Sackett. Journal of Clinical Epidemiology. https://doi.org/10.1016/j.jclinepi.2016.02.012
Ioannidis JPA, Fanelli D, Dunne DD, Goodman SN. (2015). Meta-research: Evaluation and Improvement of Research Methods and Practices. PLoS Biol https://doi.org/10.1371/journal.pbio.1002264
Naylor CD. (2018). On the Prospects for a (Deep) Learning Health Care System. JAMA. https://doi.org/10.1001/jama.2018.11103
Prasad V, Cifu A, Ioannidis JPA. (2012). Reversals of Established Medical Practices: Evidence to Abandon Ship. JAMA. http://doi.org/10.1001/jama.2011.1960
Prasad, Vinay et al. (2013). A Decade of Reversal: An Analysis of 146 Contradicted Medical Practices. Mayo Clinic Proceedings. https://doi.org/10.1016/j.mayocp.2013.05.012
Prasad, V, Cifu, A. (2015). Ending Medical Reversal: Improving Outcomes, Saving Lives. Baltimore: Johns Hopkins University Press.
Shojania KG et al. (2007). How Quickly Do Systematic Reviews Go Out of Date? A Survival Analysis. Ann Intern Med. http://annals.org/aim/fullarticle/736284/how-quickly-do-systematic-reviews-go-out-date-survival-analysis
Stoeger T, Gerlach M, Morimoto RI, Nunes Amaral LA (2018) Large-scale investigation of the reasons why potentially important genes are ignored. PLoS Biol https://doi.org/10.1371/journal.pbio.2006643
Tricoci P, Allen JM, Kramer JM, Califf RM, Smith SC. Scientific Evidence Underlying the ACC/AHA Clinical Practice Guidelines. JAMA. 2009;301(8):831–841. https://doi.org/10.1001/jama.2009.205
Topol, E. (2018). Ioannidis: Most Research Is Flawed; Let’s Fix It. Medscape. https://www.medscape.com/viewarticle/898405
Wootton, D. (2007). Bad Medicine: Doctors Doing Harm Since Hippocrates. Oxford University Press