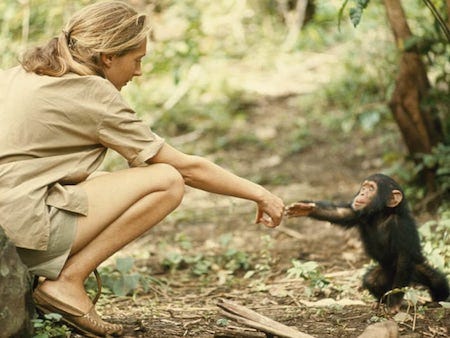
The Eyes of AI
When I was a child, I was fascinated by Jane Goodall. The images were captivating, this brave, compassionate woman quietly observing dangerous animals. It was made all the more vivid by the names she gave her subjects, like David Greybeard and Goliath.
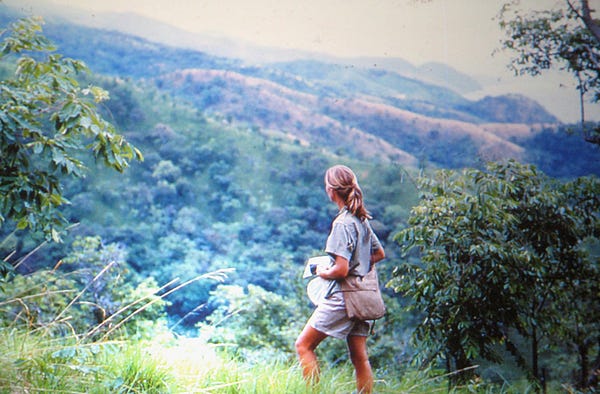
Goodall’s observations challenged orthodoxy and past conceptions. She wasn’t beyond criticism (those emotionally binding names garnered controversy). But what strikes me today is that she got out there!
What is a comparable activity for those working in artificial intelligence and machine learning? Some might say it’s the observations of domain experts. If you’re creating an application that automates a marketing task, observe how marketers behave. If it’s a medical app, consult with clinicians.
While undeniably useful and essential to questions of integration, this practice of consulting human experts seems unlike Goodall’s example. Automated systems frequently behave nothing like the manual systems they displace. And humans make use of tacit knowledge which is notoriously difficult to extract and replicate. There’s an objectivity and generality that’s needed, something quite beyond just asking the experts.
Rather, what is the purpose of AI? In essence, we need inventive solutions to our problems, the means for creating knowledge. Moreover, we want machines that create good knowledge, effective explanations of how to change the world. Science is the best example of the revolutionary knowledge creation we envision for intelligent machines.
But how do we observe knowledge creation? There are specific disciplines within philosophy that are deeply connected to activities of knowledge creation. The branches that deal with the theory of knowledge (epistemology) and the philosophy of science have an expansive reach in AI. These philosophers investigate the problem of how good knowledge is created. Yet, the dismissal of philosophy, particularly among technical people, is widespread.
“‘Computer science’ is a bit of a misnomer; maybe it should be called ‘quantitative epistemology.’” Scott Aaronson
In a joyride of a book that brings together highly philosophical and technical subject matter, Scott Aaronson comments on this attitude of “philosophical bullshitting.” He recommends a much more open and pluralistic stance. “Personally, I’m interested in results — in finding solutions to nontrivial, well-defined open problems. So, what’s the role of philosophy in that? I want to suggest a more exalted role than intellectual janitor: philosophy can be a scout. It can be an explorer — mapping out intellectual terrain for science to later move in on, and build condominiums on or whatever.”
Note Aarsonson’s pragmatism. He invites the contributions of philosophy because he’s interested in results. AI suffers its own orthodoxy and engrained conceptions. There’s a deep intellectual tradition in philosophy to challenge it. Like Goodall, many scholars are quietly observing knowledge creation in its highest forms, at a distance that brings objectivity.
Scientists used to hold this study in high regard, the illumination of problems as a natural complement to the pursuit of solutions. After a pronounced philosophical winter, is the AI community rediscovering this wisdom? Aaronson writes, “With that in mind, ‘computer science’ is a bit of a misnomer; maybe it should be called ‘quantitative epistemology.’” At the very least, maybe philosophy deserves a closer look.